Feature Fusion Via Dual-Resolution Compressive Measurement Matrix Analysis For Spectral Image Classi cation
Abstract
In the compressive spectral imaging (CSI) framework, di erent architectures have been proposed to recover high-resolution spectral images from compressive measurements. Since CSI architectures compactly capture the relevant information of the spectral image, various methods that extract classi cation features from compressive samples have been recently proposed. However, these techniques require a feature extraction procedure that reorders measurements using the information embedded in the coded aperture patterns. In this paper, a method that fuses features directly from dual-resolution compressive measurements is proposed for spectral image classi cation. More precisely, the fusion method is formulated as an inverse problem that estimates high-spatial-resolution and low-dimensional feature bands from compressive measurements. To this end, the decimation matrices that describe the compressive measurements as degraded versions of the fused features are mathematically modeled using the information embedded in the coded aperture patterns. Furthermore, we include both a sparsity-promoting and a total-variation (TV) regularization terms to the fusion problem in order to consider the correlations between neighbor pixels, and therefore, improve the accuracy of pixel-based classi ers. To solve the fusion problem, we describe an algorithm based on the accelerated variant of the alternating direction method of multipliers (accelerated-ADMM). Additionally, a classi cation approach that includes the developed fusion method and a multilayer neural network is introduced. Finally, the proposed approach is evaluated on three remote sensing spectral images and a set of compressive measurements captured in the laboratory. Extensive simulations show that the proposed classi cation approach outperforms other approaches under various performance metrics.
Collections
- Artículos de Revista [4166]
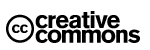