Medium range optimization of copper extraction planning under uncertainty in future copper prices
Résumé
Deterministic mine planning models along a time horizon have proved to be very effective in supporting decisions on sequencing the extraction of material in copper mines. Some of these models have been developed for, and used successfully by CODELCO, the Chilean state copper company. These models are extremely large. In this paper, we wish to consider the uncertainty in a very volatile parameter of the problem, namely, the copper price along a given time horizon. We represent the uncertainty by a multistage scenario tree. The resulting stochastic model is then converted into a mixed 0-1 Deterministic Equivalent Model using a compact representation. We first introduce the stochastic model that maximizes the expected profit along the time horizon over all scenarios (i.e., as in a risk neutral environment). We then present several approaches for risk management, in a risk averse environment. Specifically, we consider the maximization of the Value-at-Risk and several variants of the Conditional Value-at-Risk, the maximization of the expected profit minus the weighted probability of having a ``bad" scenario in the solution provided by the model, and the maximization of the expected profit subject to stochastic dominance constraints for a set of profiles given by the pairs of target profits and bounds on either the probability of not reaching them or the expected profit deficit over the targets. We present an extensive computational experience on the actual problem, by comparing the risk neutral approach, the tested risk averse strategies and the performance of the traditional deterministic approach that uses the expected value of the uncertain parameters. The results clearly show the advantage of using any risk neutral strategy over the traditional deterministic approach, as well as the advantage of using the risk averse strategy over the risk neutral one, although the plain use of the MIP solvers should be replaced by decomposition algorithms.
Colecciones
- Documentos de Trabajo [127]
Herramientas
Estadísticas
Statistiques d'usage de visualisationCitas
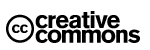