Application of AI for short-term pv generation forecast
Résumé
The efficient use of the photovoltaic power requires a good estimation of the PV generation. That is why the use of good techniques for forecast is necessary. In this research paper, Long ShortTerm Memory, Bidirectional Long Short-Term Memory and the Temporal convolutional network are studied in depth to forecast the photovoltaic power, voltage and efficiency of a 1320 Wp amorphous plant installed in the Technology Support Centre in the University Rey Juan Carlos, Madrid (Spain). The accuracy of these techniques are compared using experimental data along one year, applying 1 timestep or 15 min and 96 step times or 24 h, showing that TCN exhibits outstanding performance, compared with the two other techniques. For instance, it presents better results in all forecast variables and both forecast horizons, achieving an overall Mean Squared Error (MSE) of 0.0024 for 15 min forecasts and 0.0058 for 24 h forecasts. In addition, the sensitivity analyses for the TCN technique is performed and shows that the accuracy is reduced as the forecast horizon increases and that the 6 months of dataset is sufficient to obtain an adequate result with an MSE value of 0.0080 and a coefficient of determination of 0.90 in the worst scenarios (24 h of forecast).
Description
The authors acknowledge the support of LabTel-UFES and NiDA. Moreover, the authors would like to thank the Technological Center Support (CAT) of the University Rey Juan Carlos and the research chair Smart e2 (URJC) for their support for this research paper.
Colecciones
- Artículos de Revista [4697]
Herramientas
Estadísticas
Statistiques d'usage de visualisationCitas
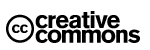